Subscribe to Our Newsletter
Sign up for our bi-weekly newsletter to learn about computer vision trends and insights.
What is Object Tracking and Re-Identification
by
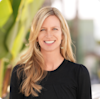
Kathleen Siddell
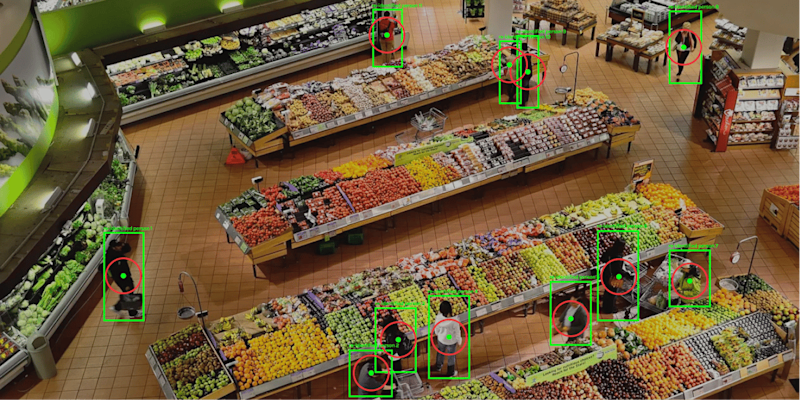
Subscribe to Our Newsletter
Sign up for our bi-weekly newsletter to learn about computer vision trends and insights.
Kathleen Siddell