Subscribe to Our Newsletter
Sign up for our bi-weekly newsletter to learn about computer vision trends and insights.
The Guide to AI-Powered Video Analytics
June 1, 2022 by
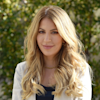
Liz Oz
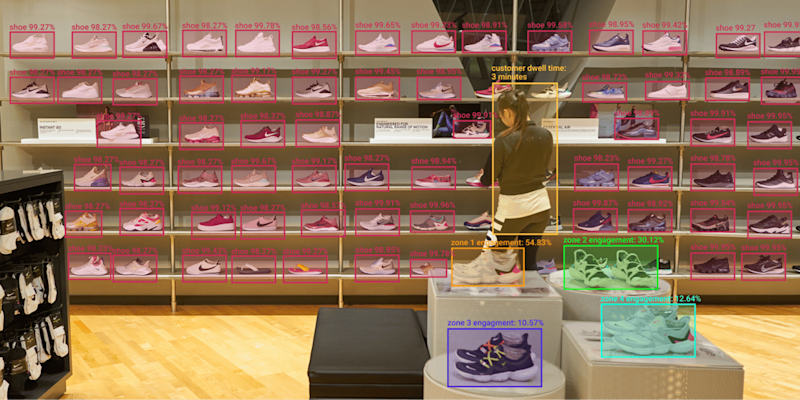
Subscribe to Our Newsletter
Sign up for our bi-weekly newsletter to learn about computer vision trends and insights.
Liz Oz